I'm sure all glider pilots in Australia have either said or heard this. But what is actually behind this statement? Do La Niña and El Niño really significantly affect gliding weather? If yes, does it make a difference whether you're up in Queensland or down in South Australia? And what other weather systems influence gliding conditions? In this blog post, I aim to address these questions and perform statistical analysis to answer which weather influences impact gliding conditions across Australia. This work is a collaboration with SkySight.
Too lazy to read the entire blog post and just want an answer? Go straight to the summary table.
Some basic climatology
Let's start with a little bit of background on climatology. the weather is incredibly important for glider pilots, as we rely on rising air to stay airborne. Thankfully, we don't need to understand the details of how weather systems influence gliding conditions, as we have SkySight that tells us whether the conditions are suitable for long flights or not. In this blogpost, we want to examine some of the climate influences and how they affect gliding conditions over longer periods.
In Australia, the weather is affected by a number of climate influences. The one that is probably most known and talked about among glider pilots is the El Niño-Southern Oscillation (ENSO). Generally speaking, El Niño is associated lower rainfall in Australia and La Niña with higher rainfall.
Other climate drivers that influence Australian weather are the Southern Annular Mode (SAM) and the Dipole Mode Index (DMI), which measures the Indian Ocean Dipole (IOD), and you can read more about them here and in the next section. Here's a nice map showing the main climate influences that affect the weather in Australia:
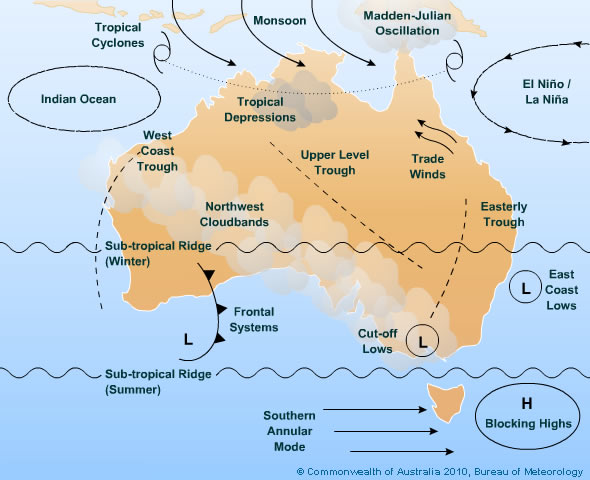
But how do these influence gliding conditions? Does ENSO really have such a significant impact? And how is each of the states impacted by the different climate influences?
In this blog post, I will perform statistical analyses to investigate whether there is a significant relationship between three of the weather systems that affect Australia and the mean points of the top 250 flights over any three months period. Please note that there is not enough data to draw a definite conclusion! Additionally, there are some other weather systems that influence the weather across Australia.